Data have long been a critical asset for organizations, businesses, and governments and their analysis is of major importance for every stakeholder in order to be able to handle and extract value and knowledge from these data. The advances in the fields of IoT, cloud computing, edge computing and mobile computing have led to the rapidly increasing volume and complexity of data, thus the concept and term of Big Data have experienced enormous interest and usability over the last decade. The spectacular growth in the creation, storage, sharing and consumption of data during the last decade indicates the need for modern organizations to fuse advanced analytical techniques with Big Data in order to deal with them and to get significant value from them. Hence, Big Data and analysis of them facilitate personalised healthcare and risk assessment, therefore clinicians are able to identify patients who are eligible for appropriate treatments, which results in savings of time and cost. R&D on personalised healthcare makes diagnostics smarter and more targeted; like in the case of Cancer, while early identification and personalised treatments can help in the design of improved screening programs and can also allow people to live longer, healthier and more productive lives. The ability to identify which preventative measure and intervention is delivering the desired impact can massively help in the development of new diagnostic and treatment regimes.
A recent survey has estimated the global market for Big Data at US $70.5 Billion in the year 2020, which is further projected to reach the size of US $86.1 Billion by 2024, growing at a CAGR of 5.25% [1]. Especially when it comes to the healthcare domain, the successful exploration and interpretation of all these data play a vital role. Healthcare data is available in different forms (e.g. images, signals and wavelengths). All these data may derive from different healthcare stakeholders (i.e. patients themselves, healthcare professionals, etc.), where more and more stakeholders place data demands on other stakeholders, and many healthcare organizations find themselves overwhelmed with data, but lacking truly valuable information. At the same time, due to the improvement in the automatic collection of data from medical devices and systems, researchers and analysts can monitor data or information that can be accessed in electronic configuration. On top of that, a crucial role in the huge expansion of healthcare data plays the wearable devices. The number of connected wearable devices in 2020 reached a total of 835.0 million wearable devices worldwide, resulting in a huge increase from the 593.0 million devices that were connected in 2018, being estimated to reach 1105 million devices until 2022 [2]. Hence, it becomes clear that this number is gradually increasing with every passing year, resulting into numerous heterogeneous devices being connected to the healthcare world, producing huge amounts of data.
Moreover, the term of Big Data defines a two-fold meaning in these data. On one hand, it describes a change in the quality and type of data that modern healthcare organizations dispose of, which has potential impacts throughout the entire healthcare domain and stakeholders. While in the other hand it describes a massive volume of both structured and unstructured data that is so huge and complicated to be processed using traditional database and software techniques. On top of this, unstructured data can be defined as data that do not comfort in predefined data models and traditional structures that can be stored in relational databases. Data generated by medical reports, medical advice, texts in questionnaires or even posts on modern social networks are such types of unstructured data and their main characteristic is that they include information that is not arranged according to a predefined data model or schema. Therefore, these types of data are usually difficult to manage, and as a result analyzing, aggregating, and correlating them in order to extract valuable information and knowledge is a challenging task. Hence, deriving value and knowledge from this type of data based on the analysis of their semantics, meanings and syntactic is of major importance. Data interoperability is the ability to merge data without losing meaning and is realized as a process of identifying the structural, syntactical, and semantic similarity of data and datasets and turn them into interoperable domain-agnostic ones. In practice, data are said to be interoperable when it can be easily reused and processed in different applications, allowing different information systems to work together and share data and knowledge. Hence, Semantic Interoperability is a key enabler for the healthcare professionals in order to enhance the exploitation of Big Data and to better understand data, by extracting and taking into account parameters and information for their patients that they were not aware of, thus creating more efficient and effective treatments, medical advices and even risk assessment strategies. The latter demonstrates the need for the modern stakeholders in the healthcare domain to implement techniques, mechanisms, and applications that focus their operations on the concept of data interoperability and more specific on Semantic Interoperability, for offering more precise and personalised prevention & intervention measures, higher experience for patients’ health monitoring, risk assessment and personalised decision support.
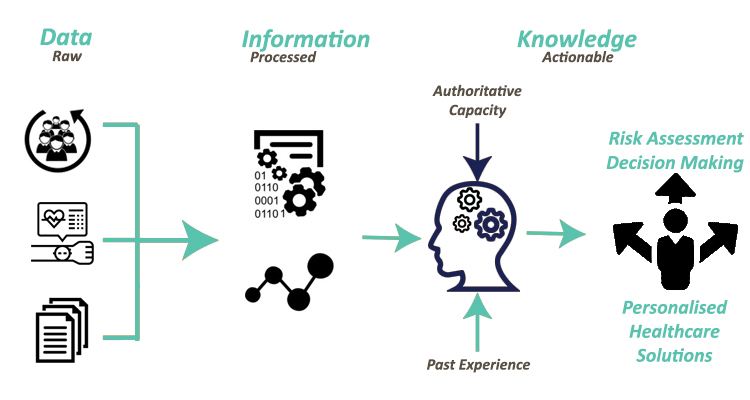
At the same time, the volume and continuous growth of the produced data, whether in the form of real-time data or stored data, in various relational and non-relational databases, has led the scientific and business communities to develop sophisticated Big Data applications based on the utilization of techniques and methods from the field of Artificial Intelligence (AI). Undoubtedly, progress in making the computer systems more human-friendly requires the inclusion of Natural Language Processing (NLP) techniques, a subfield of the modern area of AI, as integral means of a wider communication interface. NLP leverages linguistics and computer science to make human language intelligible to machines. Therefore, NLP has been used in several applications and domains to provide enhanced approaches for generating and understanding the natural languages of humans. Speech recognition, topic detection, opinion analysis, and behavior analysis are only a few of such applications and approaches. However, it can be used in concert with text mining to provide healthcare professionals with new filtering systems and more comprehensive analysis tools. In addition, NLP can be utilized as an aide to extract entities and to develop controlled vocabularies, especially enterprise schema that represents the classification of a proprietary content set, which can be further utilized for creating proper ontologies.
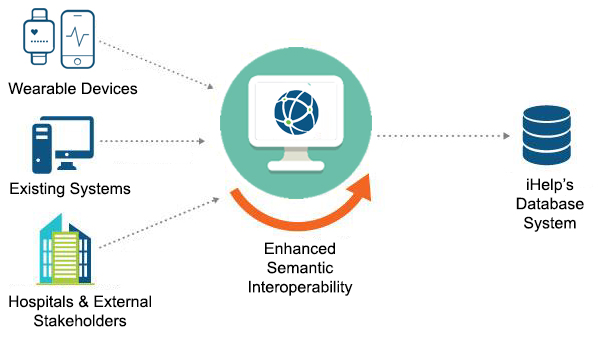
To this end, the iHelp project seeks to design and provide a novelty framework and approach that will utilize NLP and other AI techniques and tools, such as Neural Networks, and integrates them with Semantic Web technologies, such as controlled vocabularies and ontologies, for achieving Enhanced Semantic Interoperability in the healthcare domain. Through this proposed framework, on one hand, iHelp seeks to convert the clinical information into meaningful data so that healthcare systems communicate effectively, facilitating the medical and nursing healthcare personnel to reach efficient clinical decisions. While in the other hand, iHelp will be able to analyse user-generated content on social media platforms and on wearable devices in order to unlock behavioural trends and reveal lifestyle information that can fuel the development of targeted interventions.