The concept of Digital Twins was designed in 2002 by Michael Grieves [1], initially, to serve as a tool in the Product Life Management (PLM) process, that would help optimize the production and maintenance process.
There are three levels in which that can be achieved, namely:
- The Digital Model: does not automatically perform data transfer from the physical model to the digital one. Furthermore, any change in the state of the physical or digital model has no direct consequences for the other one.
- Digital Shadow: This differs from the digital model because it provides an automatic flow of information from the digital side (shadow). Changes in the physical object can interfere with the digital one, but not vice versa;
- Digital Twin: There is a flow of information between a physical asset and a digital asset and vice versa. As a shadow, the object can control the digital, but in this case, the digital also interferes with the object. This complete mutual flow is what creates a digital twin.
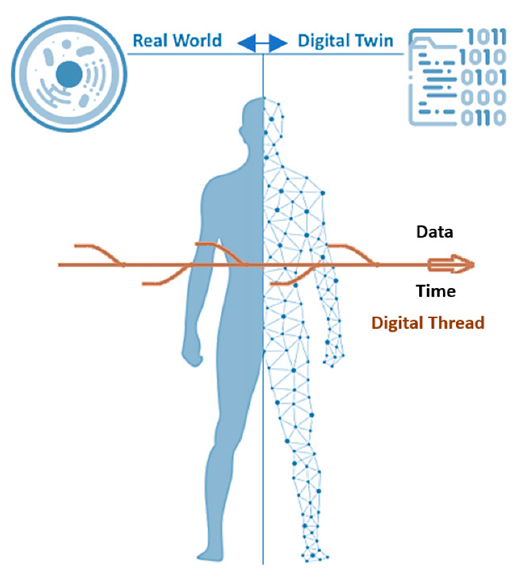
One of the goals of a Digital Twin is to provide insights from a product or a heap of products, using digital visualization tools as well as using the data flow between the physical and the digital object(s).
With the rising of the Industrial Internet of Things (IIoT) [3], and Industry 4.0, the multitude of sensors and actuators in the industrial environments, a new challenge appears: how to make sense of this overwhelming information?
As the effort to grasp the actual information is decreasing [4], and the scalable cloud environments are providing this data, other challenges appear, like keeping control over the usage of a company’s data or sharing in a fair manner the data with other partners.
The concept of dataspace gained traction with several groups exploring its utility to manage data from different domains and regions within one data ecosystem. This concept is to be popularized by many groups exploiting its use to manage data across different domains and regions in a global framework data ecosystem.
For example, the Big Data Value Association (BDVA) perspective over DataSpaces is that of an ecosystem of data models, databases, ontologies, databases contract sharing, and special administrative services (as generally offered through data centers, stores, warehouses, individually or in “data lakes”), collectively with soft skills (i.e., management, social relations, business processes) [5].
In 2022, Usländer et al [6], provide potential solutions to the following specific objectives:
- How to provide a future-oriented description of both concepts: DataSpaces and Digital Twins
- To show and demonstrate a reference model for the digital twin that comprises both concepts
- To present how digital twins can benefit in the future from the development and availability of dataspaces
Several conclusions have been drawn from the above-mentioned study and we can conclude that the two concepts are imperatively connected to each other, so in the future, more projects and technologies that link both domains will be developed.
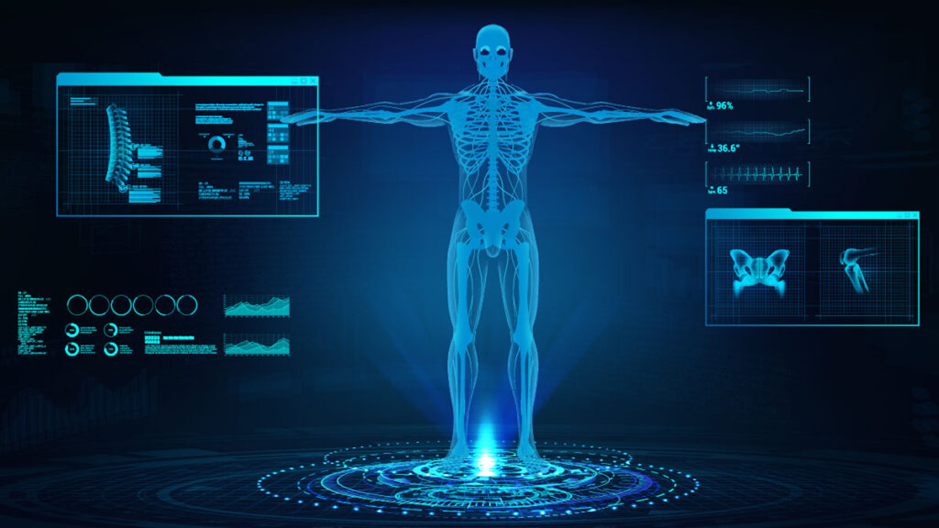
Health Digital Twins (HDTs) are defined as virtual representations (“digital twins”) of patients (“physical twins”) that consist of multimodal patient data, population data, and real-time sensing of the environment and the patients’ health conditions. Used correctly, HDTs can process random noise that affects digital twins to gain insight into the expected behavior of physical twins, providing means for innovations in medicine, clinical trials, and public health [7].
The key considerations for translating HDT research into clinical practice are: computational requirements, clinical implementation, management of data, and product monitoring.
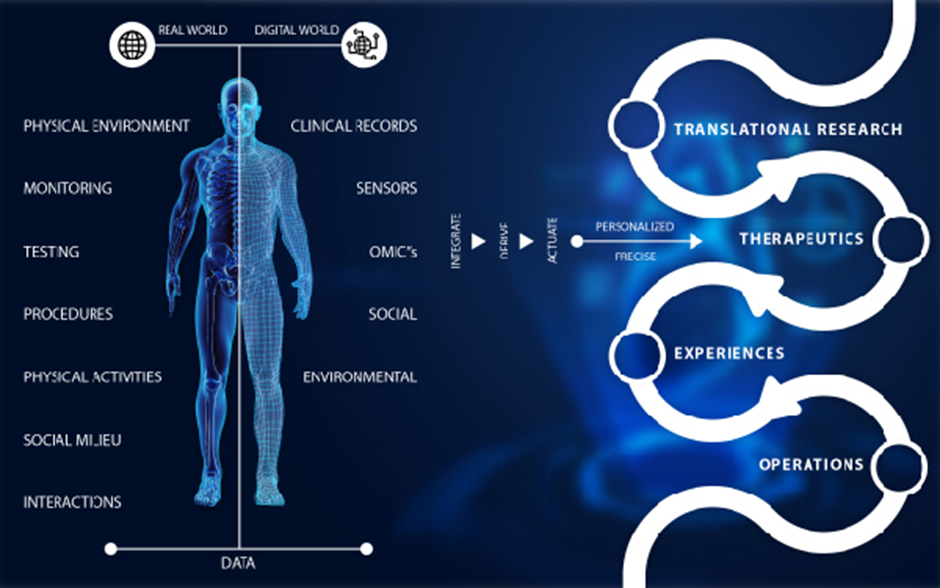
iHelp Project provides a framework designed to replicate the HDT as a representation of patients’ health status and sustain the monitoring of patient’s health by providing various tools that allow clinicians to visualize and monitor the progress of a patient.
References:
[1] Grieves, Michael. (2002). Completing the Cycle: Using PLM Information in the Sales and Service Functions, SME Management Forum, Troy, Michigan, USA.
[2] Kamel Boulos, M.N.; Zhang, P. Digital Twins: From Personalised Medicine to Precision Public Health. J. Pers. Med. 2021, 11, 745. https://doi.org/10.3390/jpm11080745
[3] Jon Hallsten, March 2017, The Industrial Internet of Things Reference Architecture, from the IIC, Hallsten Innovations Ltd [retrieved from https://www.hallsteninnovations.com/industrial-internet-things-reference-architecture-iic/?utm_source=IIoT-World.com.com&utm_medium=iiot-world.com/news/; on Jan 10th, 2023).
[4] Minerva, R.; Lee, G.M.; Crespi, N. Digital twin in the IoT context: A survey on technical features, scenarios, and architectural models. Proc. IEEE 2020, 108, 1785–1824.
[5] Scerri, S., Tuikka, T., & Lopez de Vallejoan, I. (Eds.). (April, 2019). Towards a European data sharing space: Enabling data exchange and unlocking AI potential, BDVA Position paper, https://www.bdva.eu/towards-european-data-sharing-space-bdva-position-paper.
[6] Usländer, T.; Baumann, M.; Boschert, S.; Rosen, R.; Sauer, O.; Stojanovic, L.; Wehrstedt, J.C. Symbiotic Evolution of Digital Twin Systems and Dataspaces. Automation 2022, 3, 378-399.
[7] Venkatesh, K.P., Raza, M.M. & Kvedar, J.C. Health digital twins as tools for precision medicine: Considerations for computation, implementation, and regulation. npj Digit. Med. 5, 150 (2022).
[8] Ahmad El Hajj, Towards Human-Centric Wireless Technologies: Digital Twins in Health Sector, insidetelecom: International Telecom Business Magazine, March 25, 2022 [retrieved from: https://insidetelecom.com/towards-human-centric-wireless-technologies-digital-twins-in-health-sector/, on Jan 10th, 2023]
[9] DIGITAL TWIN IN HEALTHCARE, FastStreamTechnologies [retrieved from: https://www.faststreamtech.com/wp-content/uploads/2021/03/Decision-support-of-Patient-Diagnosis-and-Treatment.jpg, on Jan 10th, 2023])