Over the past few years, the incidence of pancreatic cancer (PC) has enlarged and AI techniques have emerged as powerful tools in health research to identify potential risk factors and treatments. PC accounts for about 2% of all cancers and is related to 5% of cancer-related deaths1. Identifying pancreatic cancer is a challenging task for both clinicians and researchers. AI research has shown promise in improving early detection and diagnosis of pancreatic cancer, which could potentially improve patient outcomes.
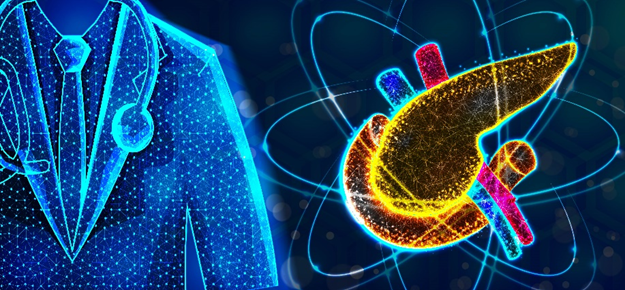
Identifying risk factors for PC
Several risk factors should be considered when conducting AI-based research on PC, including age, smoking, obesity, diabetes, chronic pancreatitis, family history, genetic mutations, race, and gender.
AI-based risk factor analysis is often performed using advanced techniques such as machine learning, deep learning, and natural language processing. Machine learning algorithms can analyze large datasets and identify complex patterns that may be missed by traditional statistical methods. These algorithms can also help identify new risk factors that were previously unknown. Deep learning algorithms, on the other hand, can analyze medical images and identify early signs of pancreatic cancer. Natural language processing can also be used to extract meaningful information from unstructured medical records, including clinical notes, pathology reports, and radiology reports.
Moreover, AI can also help in identifying the interactions between risk factors and their complex relationships. By analyzing these complex interactions, researchers can develop personalized prevention and treatment strategies that can help reduce the incidence and mortality rate of pancreatic cancer.
iHelp aims to make use of the applications of AI algorithms in combination with the benefits of mobile and wearable technologies, for improving individuals’ adherence to risk mitigation strategies, delivery of targeted health advice and even supporting lifestyle changes.
Machine learning algorithms
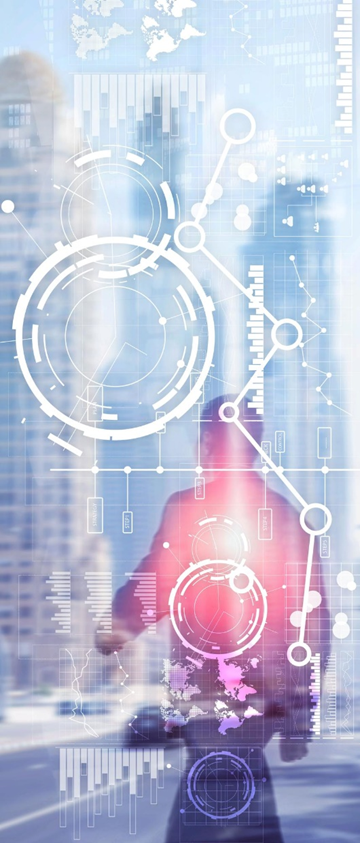
The following are some of the commonly used machine learning algorithms for risk factor analysis in pancreatic cancer research exploited also by iHelp:
Decision Trees (DT): This algorithm works by creating a tree-like model of decisions and their possible consequences. It can be used to identify the most important risk factors that lead to the development of pancreatic cancer.
Random Forest (RF): This algorithm uses a collection of decision trees and combines their predictions to make a more accurate prediction. It can be used to identify the most relevant risk factors that lead to pancreatic cancer.
Support Vector Machines (SVMs): This algorithm is used for classification tasks, where the goal is to classify a set of observations into one of two categories. SVMs can be used to identify patients at high risk of developing pancreatic cancer based on their risk factors.
To train these machine learning algorithms, the available datasets are fed containing information on various risk factors for pancreatic cancer, such as age, smoking, obesity, diabetes, and family history. The algorithms then learn to identify patterns in the data and make predictions on the risk of developing pancreatic cancer.
Once the machine learning algorithms are trained, they can be used to develop personalized prevention and treatment strategies for patients at high risk of developing pancreatic cancer. This approach can significantly improve the accuracy of risk factor analysis and enable early detection and treatment of pancreatic cancer.
[1] 1. Goral V. Pancreatic cancer: pathogenesis and diagnosis. Asian Pac J Cancer Prev APJCP. 2015;16(4):5619–5624. doi:10.7314/apjcp.2015.16.14.5619 [PubMed] [Google Scholar]